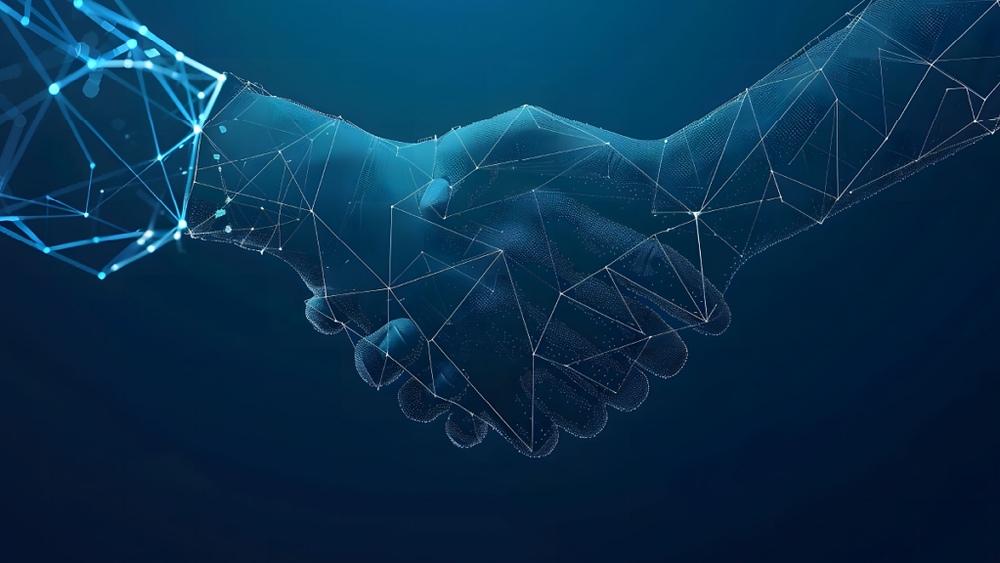
Trust Before Prompt: The Hidden Work Behind Successful AI Adoption
2
5
0
By the EHCOnomics Team
Ethical Intelligence. Built Around You.
Introduction: Trust Isn’t a Feature. It’s the Prerequisite.
The failure point in most AI adoption stories isn’t functionality. It’s psychology. Teams aren’t walking away from systems because they lack features—they’re stepping back because they lack certainty. Even the most advanced intelligence platform stalls if users can’t understand how it behaves, what it remembers, or whether it will violate the invisible expectations that shape organizational culture. Prompt training, UI walkthroughs, and use-case documents are all necessary. But none of them are foundational. If people don’t trust what the system is doing before they interact with it, the interaction never becomes meaningful.
At EHCOnomics, we build from that point of tension. Not from interface outward—but from architecture up. Because the moment a system touches a user's decision space, it’s not just offering support. It’s asking for trust. And in high-friction, high-velocity environments, that trust doesn’t come from what the system can do. It comes from what it refuses to do—retain behavior, infer preference, or operate without traceable logic. Trust isn’t a passive outcome. It’s a structural behavior.
The Real Barrier to Successful AI Adoption Is Not Complexity—It’s Confidence
Research from Deloitte in 2024 confirms what we've observed repeatedly: More than half are concerned that the widespread use of Gen AI will increase economic inequality (51%). This finding aligns with broader behavioral trends around system hesitancy. Teams don’t reject AI because they can’t understand the interface. They reject it because they can’t trace the logic. When systems produce polished outputs without visible rationale—or worse, retain data in ways users don’t expect—the result isn’t excitement. It’s withdrawal.
These aren’t irrational fears. According to a 2023 IBM study, half (57%) of CEO respondents are concerned about data security and 48% worry about bias or data accuracy—above performance, above integration, and above deployment complexity. In other words, trust outpaces even power as the defining variable of long-term AI viability. Without it, adoption is performative. People click. But they don’t commit.
A.R.T.I.’s Answer: Make Trust Observable, Not Optional
We designed A.R.T.I. (Artificial Recursive Tesseract Intelligence) from the premise that intelligence must behave with restraint before it can be trusted with responsibility. It doesn’t just work within ethical guardrails—it exposes them. Every interaction with A.R.T.I. is session-bound and forgetful. It doesn’t log prompts. It doesn’t track behavior. It doesn’t build persistent profiles. And it never trains on user sessions. The session is scoped. The context is temporary. The logic is clean.
But forgetfulness alone is not enough. Trust also requires traceability. Every output A.R.T.I. generates is accompanied by visible scaffolding: logic trees, confidence indicators, and ethical flags. This transparency transforms AI from an inference machine into a coherent thinking partner. And while most LLMs still carry hallucination rates between 10–30% depending on domain, some range to as high as 88% (as reported in Stanford’s 2024 Hallucinating Law Report), A.R.T.I. remains architected for structured interaction with under 1% error in scoped, system-based workflows. But more importantly than the number is the system’s behavior when uncertainty arises—it flags low-confidence outputs, invites human override, and never conceals ambiguity.
How Trust Changes User Behavior—Even Before It’s Taught
When trust is embedded as a design principle, behavior shifts before training begins. Users don’t require reprogramming. They require confirmation—confirmation that the system won’t violate their logic, memory, or boundaries. When that confirmation is felt, usage becomes natural. Users engage without fear of being profiled. They delegate without second-guessing the source. And they return—not because the system impressed them, but because it didn’t burden them.
The psychological overhead of using AI—especially for non-technical teams—is rarely discussed. Yet it’s often the biggest blocker. That tension doesn’t come from hard-to-use systems. It comes from systems that are ambiguous about what they’re doing, and unclear about who they’re doing it for. A system like A.R.T.I. doesn’t solve that by being “easy.” It solves it by being interpretable. And interpretation builds trust. Not through branding. Through behavior. This is Successful AI Adoption by design.
Conclusion: Don’t Assume Prompts Will Lead. Build the Trust That Lets Them Begin
AI systems that perform without explaining, retain without permission, or adapt without transparency don’t scale. They stall. Because trust is not a user journey stage—it’s the load-bearing wall. If your system doesn’t show how it thinks, users will stop trusting what it says. And if they don’t trust what it says, they won’t type the next line. That’s not resistance. It’s rational caution.
At EHCOnomics, we built A.R.T.I. not to sound intelligent—but to behave responsibly. Every design decision we’ve made—from ephemeral sessions to logic scaffolding to role-calibrated interaction frames—was built to support one thing first: structural trust. Because no matter how powerful a system is, it’s irrelevant if no one feels safe enough to use it.
Trust doesn’t follow prompts. It makes them possible.